Rui Ye / 叶锐
I am a third-year PhD candidate at Shanghai Jiao Tong University (SJTU) in Shanghai, China. Before that, I received my Bachelor degree from SJTU, ranked the first out of 150.
I am currently advised by Prof. Siheng Chen, in the MediaBrain Lab. My research interests lie in Collaborative AI and Trustworthy AI, with a particular focus on LLM-based multi-agent systems, trustworthy large language models (LLMs), and federated learning. I have previously interned at Microsoft Research Asia (MSRA) and Shanghai AI Laboratory.
I am actively seeking collaborations and opportunities as a research intern or visiting student, please feel free to contact me!!!
Email  / 
Google Scholar  / 
Github  / 
LinkedIn  / 
Twitter
|
|
📑 Publications
* denotes equal contribution, † denotes corresponding author, see full list in Google Scholar, some are highlighted.
|
2025
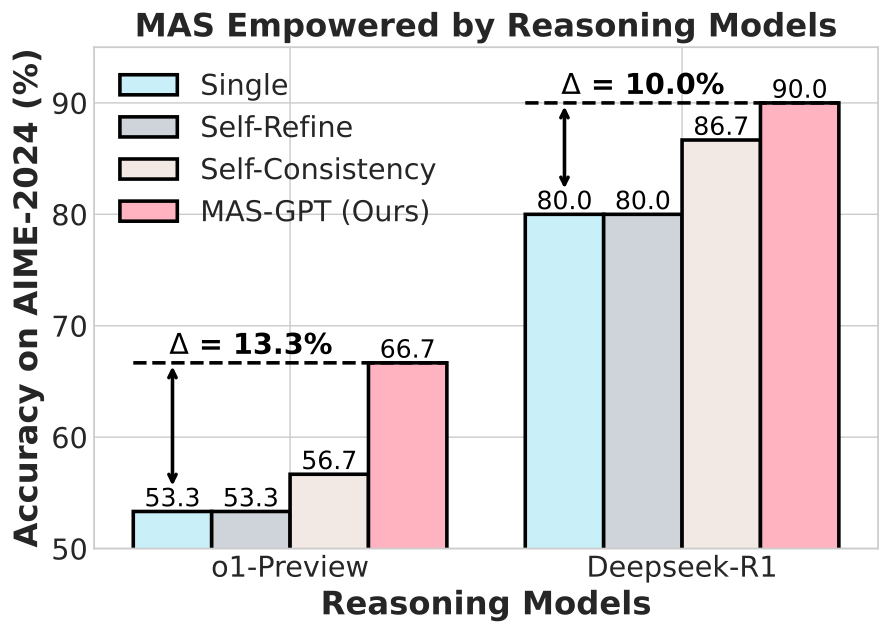
|
MAS-GPT: Training LLMs to Build LLM-based Multi-Agent Systems
Rui Ye, Shuo Tang, Rui Ge, Yaxin Du, Zhenfei Yin, Siheng Chen†, Jing Shao†
Preprint, 2025
arXiv / BibTeX
This paper proposes to formulate the process of building LLM-based multi-agent systems (MAS) as a generative task, making it as simple as querying ChatGPT. We design a dataset construction pipeline and train MAS-GPT, a 32B LLM capable of generating an executable MAS give any specific query. Results demonstrate MAS-GPT’s simplicity, cost-efficiency, and generality.
|
|
Incentivizing Inclusive Contributions in Model-Sharing Markets
Enpei Zhang*, Jingyi Chai*, Rui Ye*, Yanfeng Wang, Siheng Chen†
Nature Communications
OpenReview / BibTeX
This paper proposes inclusive and incentivized personalized federated learning (iPFL), which incentivizes data holders with diverse purposes to collaboratively train personalized models without revealing raw data.
|
2024
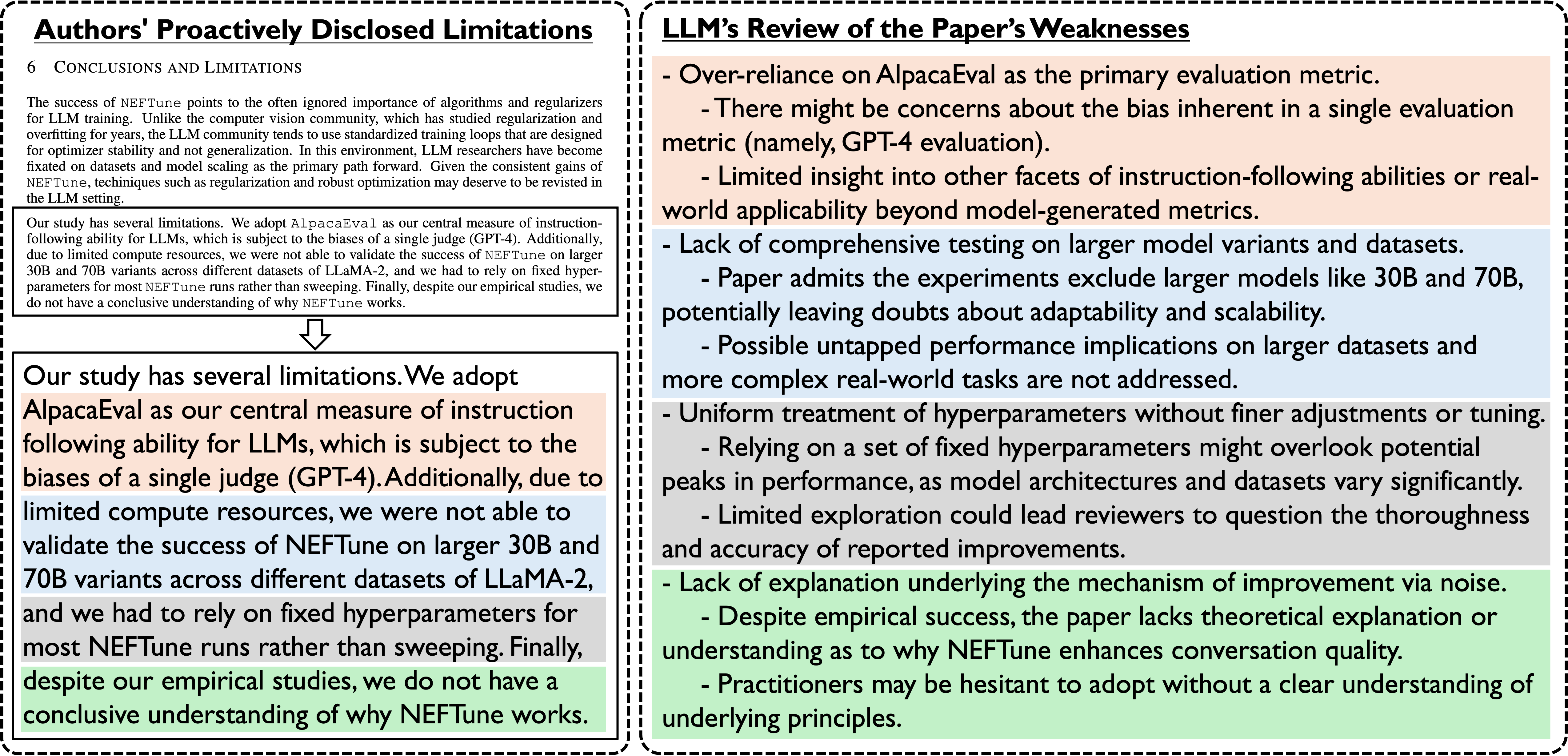
|
Are We There Yet? Revealing the Risks of Utilizing Large Language Models in Scholarly Peer Review
Rui Ye*, Xianghe Pang*, Jingyi Chai, Jiaao Chen, Zhenfei Yin, Zhen Xiang, Xiaowen Dong, Jing Shao, Siheng Chen†
Preprint, 2024
arXiv / BibTeX / Project Page
Given that LLMs are being integrated into peer review, in this study, we comprehensively reveal the vulnerabilities of LLM-generated reviews by focusing on (explicit and implicit) manipulation and inherent flaws (hallucination and bias). Our findings underscore that we are not yet ready for widespread adoption, emphasizing the need for punitive measures, detection techniques, and robust safeguards.
|
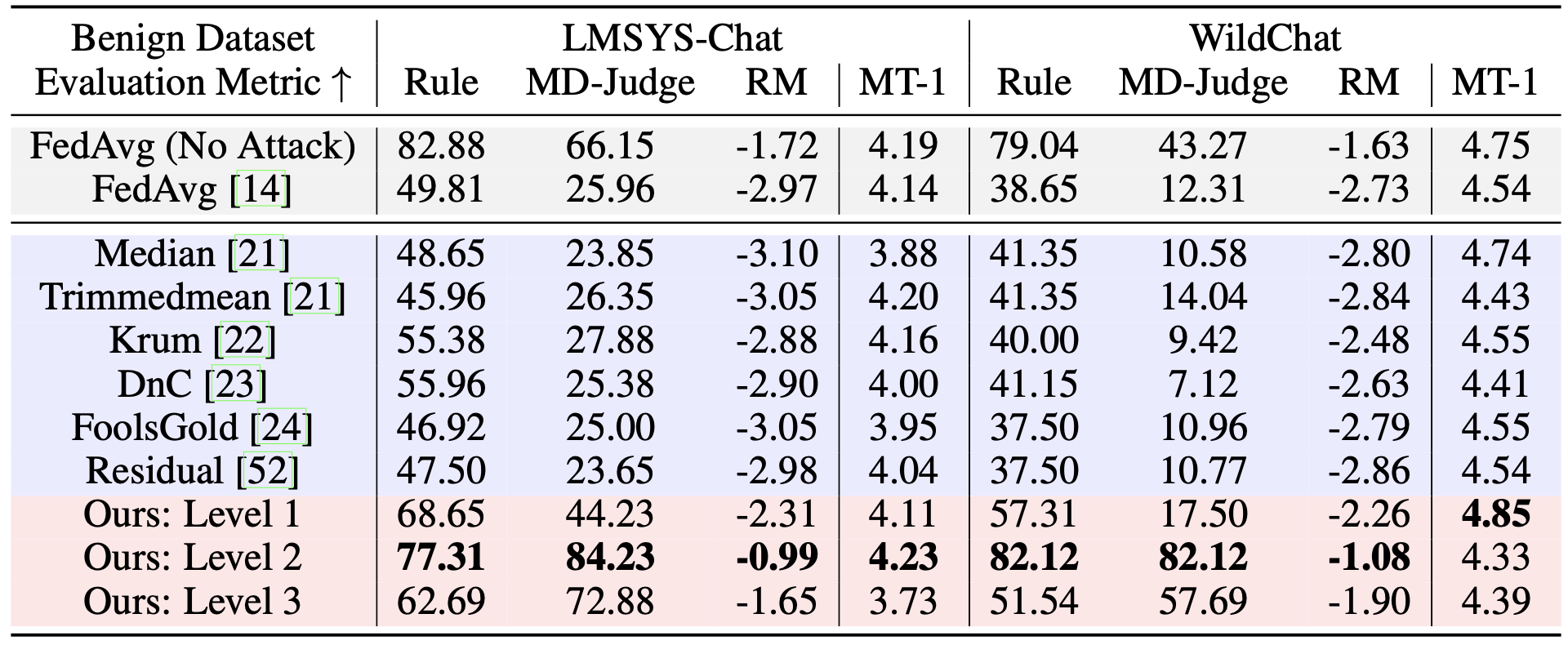
|
Emerging Safety Attack and Defense in Federated Instruction Tuning of Large Language Models
Rui Ye*, Jingyi Chai*, Xiangrui Liu*, Yaodong Yang, Yanfeng Wang, Siheng Chen†
International Conference on Learning Representations (ICLR), 2025
arXiv / BibTeX
This paper for the first time reveals the vulnerability of safety alignment during federated instruction tuning by proposing a simple safety attack method. While many existing FL defense methods fail to defend against such attack, we propose a post-hoc defense method that automatically and effectively enhances the safety alignment of LLMs.
|
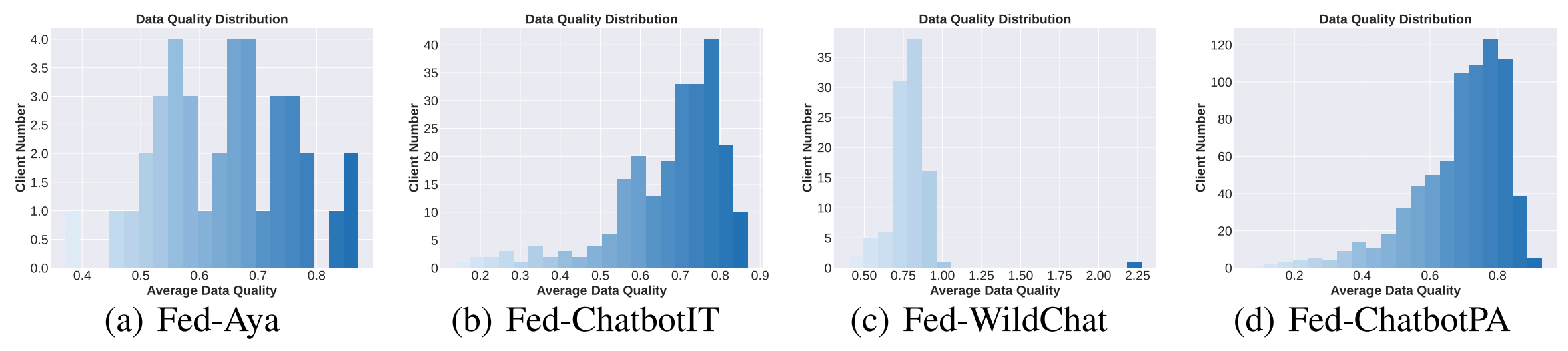
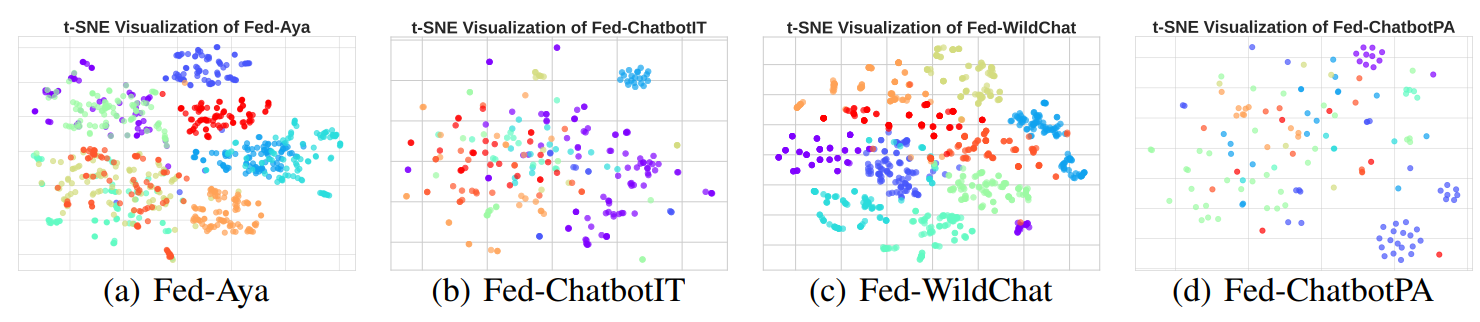
|
FedLLM-Bench: Realistic Benchmarks for Federated Learning of Large Language Models
Rui Ye*, Rui Ge*, Xinyu Zhu, Jingyi Chai, Yaxin Du, Yang Liu, Yanfeng Wang, Siheng Chen†
Conference on Neural Information Processing Systems (NeurIPS), 2024
arXiv / BibTeX / Code
This paper proposes the first realistic benchmark for federated learning of large language models, termed FedLLM-Bench. It encompasses 3 datasets for instruction tuning task and 1 dataset for preference alignment task, which exhibit diversities in language, quality, quantity, instruction, length, embedding, and preference.
|
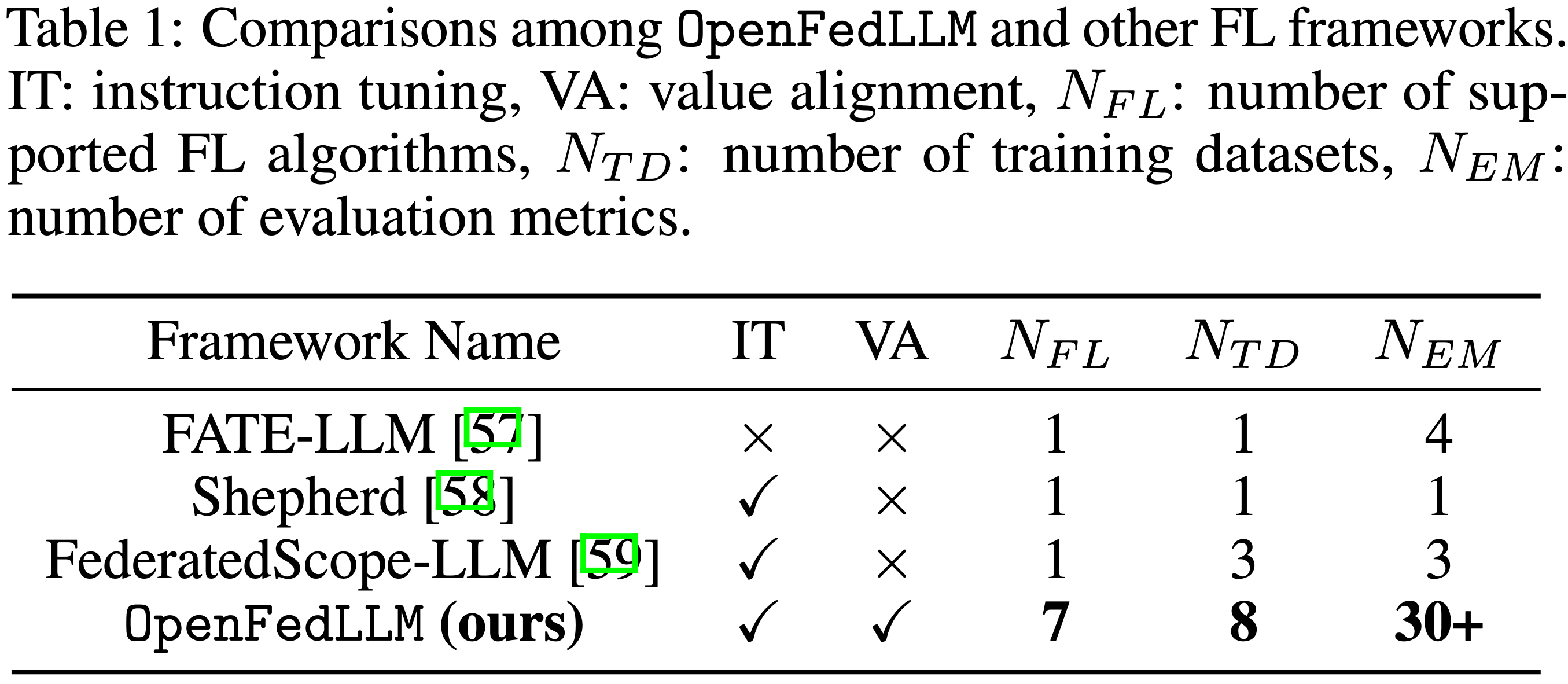
|
OpenFedLLM: Training Large Language Models on Decentralized Private Data via Federated Learning
Rui Ye, Wenhao Wang, Jingyi Chai, Dihan Li, Zexi Li, Yinda Xu, Yaxin Du, Yanfeng Wang, Siheng Chen†
Conference on Knowledge Discovery and Data Mining (KDD), 2024
ICLR AGI Workshop and DPFM Workshop, 2024
arXiv / ACM / BibTeX / Code
This paper proposes OpenFedLLM for training large language models on decentralized private data via federated learning, which covers instruction tuning, value alignment, 7 FL algorithms, 8 training datasets, and 30+ evaluation metrics. Based on OpenFedLLM, we conduct a comprehensive empirical study, provide insights, and point out future directions.
|
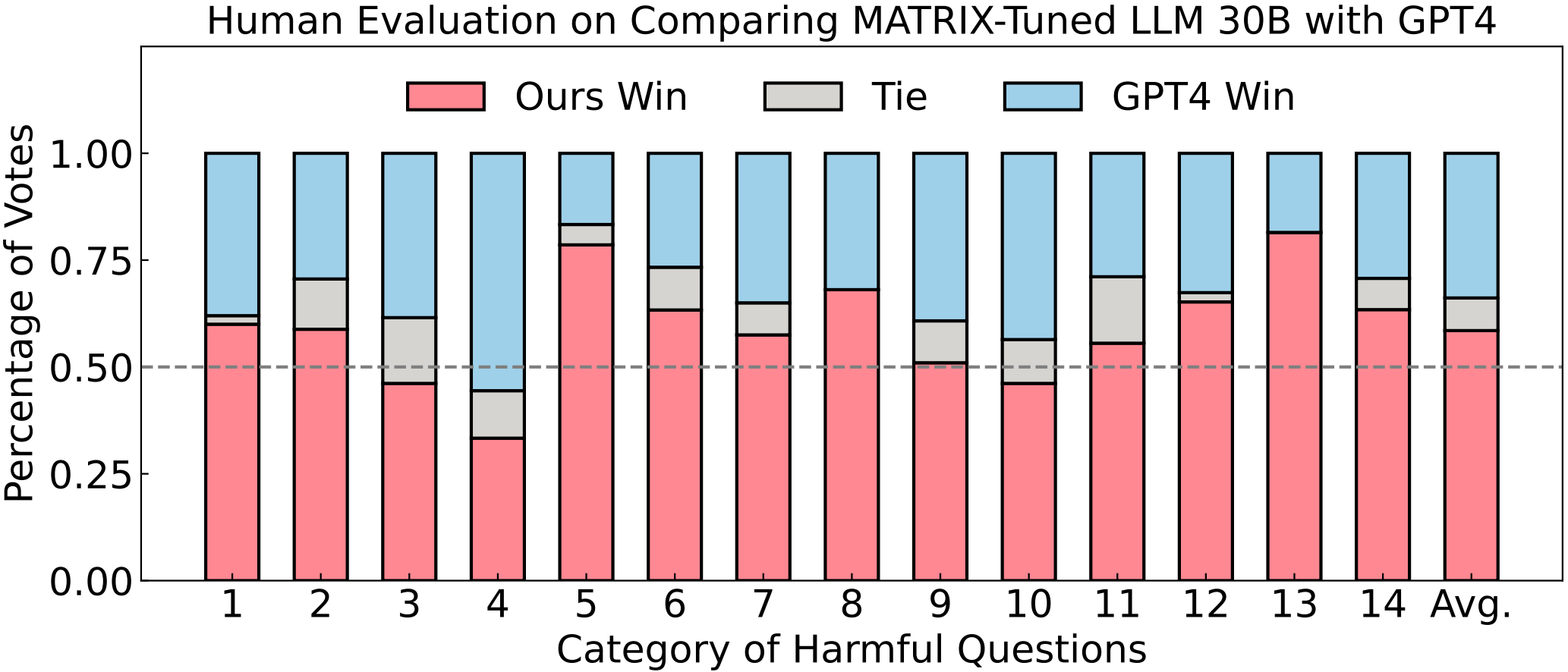
|
Self-Alignment of Large Language Models via Monopolylogue-based Social Scene Simulation
Xianghe Pang*, Shuo Tang*, Rui Ye*, Yuxin Xiong, Bolun Zhang, Yanfeng Wang, Siheng Chen†
International Conference on Machine Learning (ICML), Spotlight, 2024
ICLR AGI Workshop, Oral, 2024
arXiv / OpenReview / BibTeX / Project / Code
This paper proposes to self-align large language models via social scene simulation, which is powered by our proposed simulator called MATRIX. Human evaluations show that our aligned 13/30B LLMs can outperform GPT-4 on value alignment.
|
|
On the Vulnerability of Safety Alignment in Open-Access LLMs
Jingwei Yi*, Rui Ye*, Qisi Chen, Bin Zhu, Siheng Chen†, Defu Lian, Guangzhong Sun, Xing Xie, Fangzhao Wu†
Findings of the Association for Computational Linguistics (ACL), 2024
Paper / BibTeX
This paper unreveals the vulnerability of value alignment in aligned open-source LLMs by proposing a series of efficient attack methods (i.e., reverse alignment). Experiments show that simple fine-tuning can significantly compromise the alignment of the LLMs.
|
2023
|
Fake It Till Make It: Federated Learning with Consensus-Oriented Generation
Rui Ye, Yaxin Du, Zhenyang Ni, Siheng Chen†, Yanfeng Wang
International Conference on Learning Representations (ICLR), 2024
Paper / BibTeX / Code
This paper proposes to more fundamentally handle data heterogeneity from the perspective of data, which is achieved by extracting consensus data from the global model to complement clients' heterogeneous data.
|
|
FedDisco: Federated Learning with Discrepancy-aware Collaboration
Rui Ye, Mingkai Xu, Jianyu Wang, Chenxin Xu, Siheng Chen†, Yanfeng Wang
International Conference on Machine Learning (ICML), 2023
arXiv / BibTeX / PMLR / Code
Based on our empirical and theoretical observations, we propose to aggregate models based on both dataset size and a defined discrepancy value.
|
|
Personalized Federated Learning with Inferred Collaboration Graphs
Rui Ye*, Zhenyang Ni*, Fangzhao Wu, Siheng Chen†, Yanfeng Wang
International Conference on Machine Learning (ICML), 2023
PMLR / BibTeX / Code
We propose a pFedGraph algorithm to promote more collaboration between clients with more similar data distributions.
|
|
FedFM: Anchor-based Feature Matching for Data Heterogeneity in Federated Learning
Rui Ye, Zhenyang Ni, Chenxin Xu, Jianyu Wang, Siheng Chen†, Yanfeng Wang
IEEE Transactions on Signal Processing (TSP), 2023
Paper / IEEE / BibTeX / Code (PyTorch, PaddlePaddle, MindSpore)
We propose to align category-wise feature spaces of clients in FL, which achieves pleasant performance with theoretical convergence guarantee.
|
|
Degree: Bachelor
Period: 2018.09 - 2022.06
Major: Information Engineering (AI Class)
GPA: 3.94/4.3 (ranked 1st out of 150)
|
- National Scholarship for PhD Students, 2024
- National Scholarship for Undergraduates, 2020 (2 out of 150)
- Shanghai Outstanding Graduates, 2022
- Samsung Scholarship, 2023 (only one awardee)
- Mathematical Contest in Modeling, Finalist, 2021 (<1%)
- Shanghai Jiao Tong University Wenjun Wu AI Class, 2022 (16 are selected)
- Shanghai Jiao Tong University Xu Zhang Academician Scholarship, 2022 (3 out of 150)
- Shanghai Jiao Tong University Ceyear Scholarship, 2021
- Shanghai Jiao Tong University Fujian Alumni Association Scholarship, 2019 (the youngest awardee)
- Shanghai Jiao Tong University Class B Scholarship, 2019&2020&2021
|
Review:
- 2025: ICLR, ICML, CPVR, AAAI
- 2024: ICLR, ICML, NeurIPS Main, NeurIPS Data, ICASSP
- 2023: NeurIPS Main
|
Life:I love playing basketball / listening rap music / travelling.
|
|